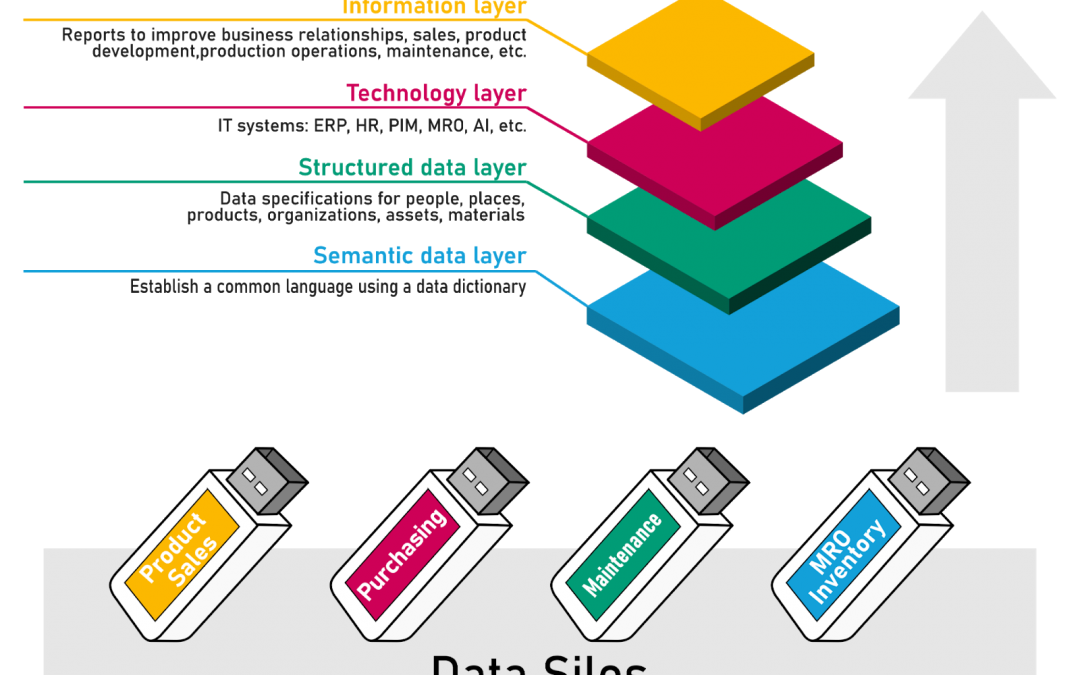
Why you should launch a data management program – A (data) message to the C-suite
Why you should launch a data management program – A (data) message to the C-suite
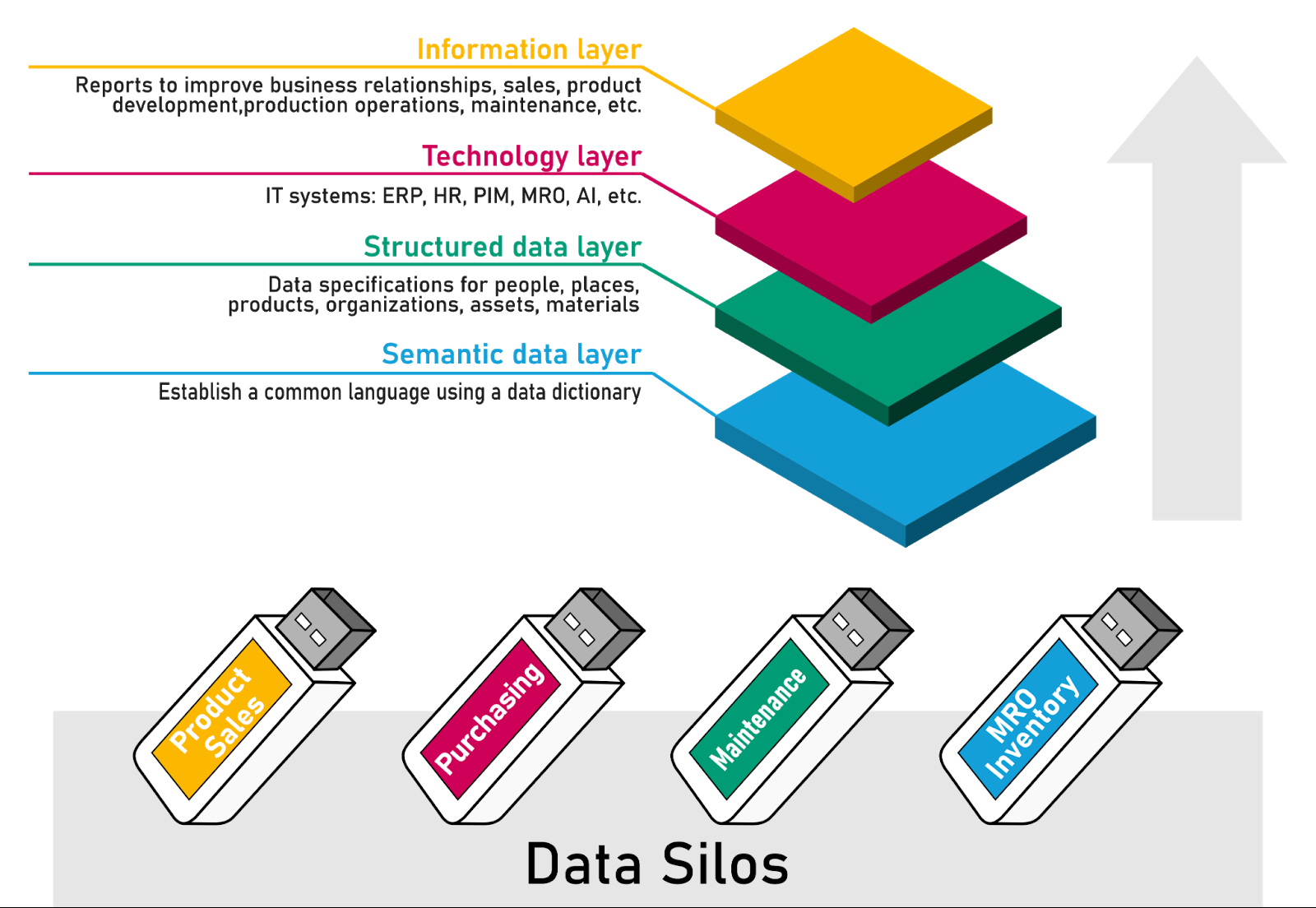
If you are an executive in a rapidly growing organization whose stated mission is “to be the most successful business in the world” you will be aware that rapid growth brings a lot of excitement, and that this very growth changes the nature of the way the company operates.
Instinctive understanding of the business becomes more challenging as more operating sites and businesses are added to the group. Executives can no longer rely solely on their knowledge of individual sites, and as the business grows, they rely more on reports compiled from the different businesses to keep a grip on the business metrics.
Any significant increase in new data brings new dynamics. Data silos multiply making it more difficult to aggregate data across departments; across sites; across regions; and across national borders. Recently acquired business units will inevitably lack a common language. Some common phrases may even have a different meaning in different parts of the organization. This lack of a common understanding makes it more likely that business opportunities will be missed.
Good quality master data is the foundation for making sound business decisions, and also for identifying potential risks to the business. Why is master data important? Master data is the data that identifies and describes individuals; organizations; locations; goods; services; processes; rules; and regulations, so master data runs right through the business.
All departments in the business need structured master data. What is often misunderstood is that the customer relationship management (CRM) system; the enterprise resource management system (ERP); the payroll system; and the finance and accounting systems are not going to fix poor master data, the software performance always suffers because of poor quality data., but the software itself will not cure that problem.
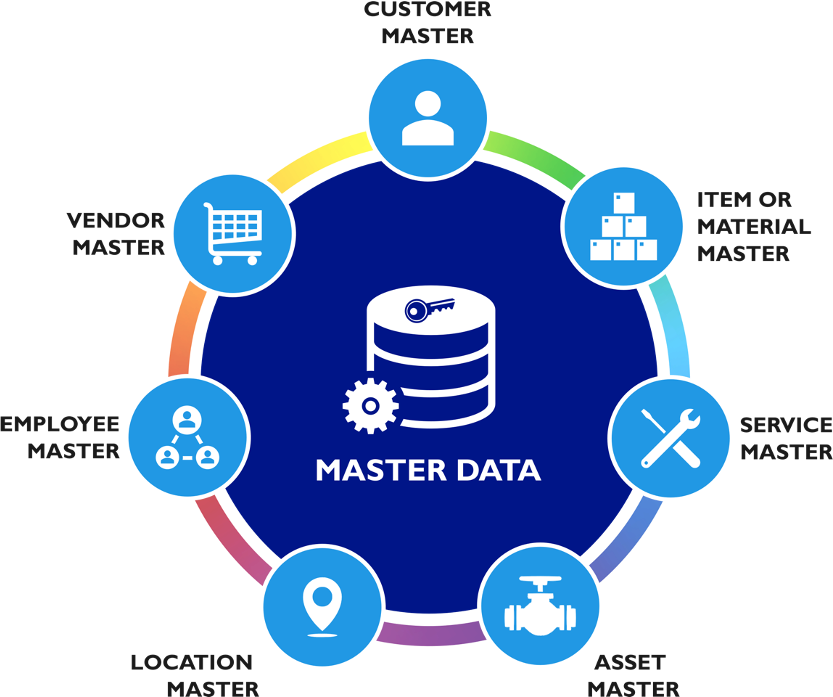
The key is to recognise what master data is not; master data is not an information technology (IT) function, master data is a business function. Improving master data is not a project. Managing master data is a program and a function that should be at the heart of the business process.
Foundational master data, that is well structured, and good quality is a necessity if your business is going to efficiently and effectively process commercial data; transaction reporting; and business activity. The reality is that well-structured, good quality, master data is also the most effective way to connect multiple systems and processes, both internally and externally.
Good quality data is the pathway to good quality information. With insight from good quality information, business leaders can identify – and no longer need to accept – the basic business inefficiencies that they know exist, but cannot pin down. For a manufacturing company making 5% profit on sales, every $50,000 in operational savings is equivalent to $1,000 000 sales. It follows then, that if you have $50,000 of efficiency savings that you can identify and implement as a result of better quality information, you have solved a million-dollar problem.
If you, as an executive, are unsure of the integrity and accuracy of the very data that is the foundation for the reports you rely on in your organization, launching a master data management program is the best course of action you can take.
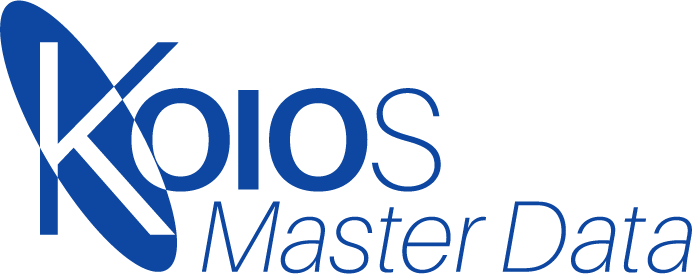
Contact us
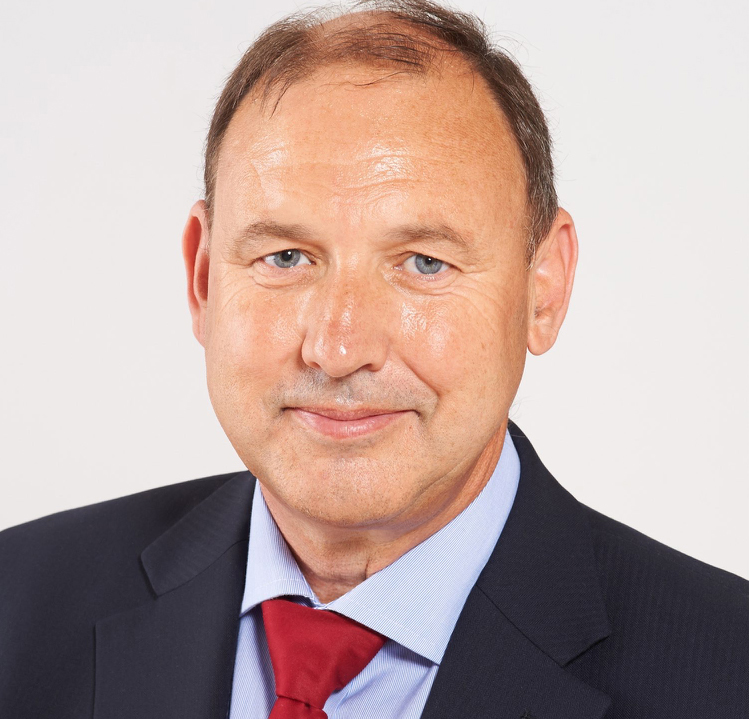
About the author
Peter Eales is a subject matter expert on MRO (maintenance, repair, and operations) material management and industrial data quality. Peter is an experienced consultant, trainer, writer, and speaker on these subjects. Peter is recognised by BSI and ISO as an expert in the subject of industrial data. Peter is a member ISO/TC 184/SC 4/WG 13, the ISO standards development committee that develops standards for industrial data and industrial interfaces, ISO 8000, ISO 29002, and ISO 22745. Peter is the project leader for edition 2 of ISO 29002 due to be published in late 2020. Peter is also a committee member of ISO/TC 184/WG 6 that published the standard for Asset intensive industry Interoperability, ISO 18101.
Peter has previously held positions as the global technical authority for materials management at a global EPC, and as the global subject matter expert for master data at a major oil and gas owner/operator. Peter is currently chief executive of MRO Insyte, and chairman of KOIOS Master Data.
KOIOS Master Data is a world-leading cloud MDM solution enabling ISO 8000 compliant data exchange